In recent years, advancements in artificial intelligence have led to the development of sophisticated multimodal models capable of understanding and processing multiple forms of data, such as text, images, and even audio. DeepSeek-VL, a state-of-the-art multimodal AI model, has garnered significant attention for its ability to seamlessly integrate visual and linguistic information to perform complex tasks. This article serves as a beginner’s guide to understanding DeepSeek-VL, breaking down its core components, architecture, practical applications, and the challenges it faces. By demystifying this cutting-edge technology, we aim to provide readers with a clear and comprehensive overview of its capabilities and potential.
Core Components and Functionality
DeepSeek-VL is built on a modular architecture that allows it to process and generate both text and images. At its core, the model consists of three primary components: a multimodal encoder, a text decoder, and an image decoder. The multimodal encoder is responsible for combining visual and textual inputs into a unified representation, enabling the model to understand the relationships between words and images. This encoder leverages self-attention mechanisms to capture long-range dependencies in both modalities, ensuring that the model can contextualize information effectively.
The text decoder is specialized for generating human-like text based on the multimodal inputs it receives. It works similarly to traditional language models but with the added capability of incorporating visual context. This allows the model to provide more accurate and relevant responses when faced with tasks that require understanding images or visual descriptions. On the other hand, the image decoder is designed to generate visual content, such as images or diagrams, based on textual prompts or combinations of text and images.
One of the most notable features of DeepSeek-VL is its ability to perform cross-modal generation. This means that users can input text to generate images or input images to generate text, making the model highly versatile for a wide range of applications. The model is also pre-trained on large-scale datasets that include diverse examples of text-image pairs, enabling it to generalize well across different domains and tasks.
Understanding the Architecture and Data Processing
The architecture of DeepSeek-VL is designed to handle the unique challenges of multimodal processing. It uses a transformer-based architecture, which is well-suited for capturing complex relationships between different types of data. The model employs vision and language encoders to process images and text separately before combining them into a shared latent space. This shared space allows the model to align visual and linguistic representations, enabling it to understand how words and images relate to each other.
When processing data, DeepSeek-VL first tokenizes the input text into subwords and converts images into a sequence of visual tokens using techniques such as discrete visual representation learning. These tokens are then passed through the respective encoders, which generate embeddings that capture the semantic meaning of the input. The embeddings are combined using cross-attention mechanisms, which allow the model to focus on relevant parts of the input when processing each modality.
A key innovation in DeepSeek-VL is its use of contrastive learning during pre-training. This approach helps the model learn to align visual and textual embeddings by minimizing the distance between corresponding text-image pairs while maximizing the distance between non-corresponding pairs. This training strategy enhances the model’s ability to understand the semantic connections between different modalities and improves its performance on downstream tasks.
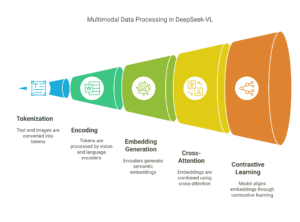
Exploring Practical Applications Across Industries
DeepSeek-VL’s multimodal capabilities make it a valuable tool for a wide range of industries. In healthcare, the model can be used to analyze medical images, such as X-rays or MRIs, and generate detailed diagnostic reports. It can also assist in drug discovery by visualizing molecular structures and providing textual descriptions of their properties. These applications have the potential to significantly enhance the speed and accuracy of medical diagnoses and research.
In the education sector, DeepSeek-VL can serve as a powerful teaching aid. For example, it can generate visual explanations of complex concepts, such as historical events or scientific principles, to help students better understand and retain information. The model can also create personalized learning materials, such as interactive diagrams or customized study guides, tailored to individual students’ needs.
The retail and e-commerce industries can also benefit from DeepSeek-VL’s capabilities. The model can be used to generate product descriptions and high-quality product images, reducing the time and cost associated with content creation. Additionally, it can enhance customer experiences by providing visual recommendations based on textual searches or generating virtual product demonstrations to help customers make informed purchasing decisions.
Navigating Challenges and Limitations
Despite its impressive capabilities, DeepSeek-VL faces several challenges that limit its adoption and effectiveness. One of the primary concerns is the quality and availability of training data. Multimodal models require large-scale datasets that include diverse examples of text-image pairs. However, obtaining such data can be difficult, especially in specialized domains where data may be scarce or sensitive. Additionally, ensuring that the training data is balanced and free from biases is crucial to avoid perpetuating harmful stereotypes or inaccuracies.
Another challenge is the computational complexity of training and deploying multimodal models. DeepSeek-VL requires significant computational resources to process and generate high-quality outputs, making it inaccessible to organizations with limited budgets or infrastructure. Furthermore, the model’s inference speed can be a bottleneck in real-time applications, where quick responses are critical.
Finally, the ethical implications of using multimodal AI models like DeepSeek-VL cannot be overlooked. The ability to generate realistic images and convincing text raises concerns about misinformation, deepfakes, and intellectual property infringement. Addressing these issues requires careful consideration of ethical guidelines and the implementation of safeguards to prevent misuse.
DeepSeek-VL represents a significant leap forward in the development of multimodal AI, offering unprecedented capabilities for processing and generating text and images. By understanding its core components, architecture, and practical applications, users can unlock its potential to drive innovation across industries. However, navigating the challenges and limitations of this technology is equally important to ensure its responsible and effective use. As multimodal AI continues to evolve, models like DeepSeek-VL will play a pivotal role in shaping the future of artificial intelligence and its applications in our daily lives.
Comments are closed.